However, the MMOD implementation in dlib used HOG feature extraction followed by a single linear filter. This means it's incapable of learning to detect objects that exhibit complex pose variation or have a lot of other variability in how they appear. To get around this, users typically train multiple detectors, one for each pose. That works OK in many cases but isn't a really good general solution. Fortunately, over the last few years convolutional neural networks have proven themselves to be capable of dealing with all these issues within a single model.
So the obvious thing to do was to add an implementation of MMOD with the HOG feature extraction replaced with a convolutional neural network. The new version of dlib, v19.2, contains just such a thing. On this page you can see a short tutorial showing how to train a convolutional neural network using the MMOD loss function. It uses dlib's new deep learning API to train the detector end-to-end on the very same 4 image dataset used in the HOG version of the example program. Happily, and very much to the surprise of myself and my colleagues, it learns a working face detector from this tiny dataset. Here is the detector run over an image not in the training data:
I expected the CNN version of MMOD to inherit the low training data requirements of the HOG version of MMOD, but working with only 4 training images is very surprising considering other deep learning methods typically require many thousands of images to produce any kind of sensible results.
The detector is also reasonably fast for a CNN. On the CPU, it takes about 370ms to process a 640x480 image. On my NVIDIA Titan X GPU (the Maxwell version, not the newer Pascal version) it takes 45ms to process an image when images are processed one at a time. If I group the images into batches then it takes about 18ms per image.
To really test the new CNN version of MMOD, I ran it through the leading face detection benchmark, FDDB. This benchmark has two modes, 10-fold cross-validation and unrestricted. Both test on the same dataset, but in the 10-fold cross-validation mode you are only allowed to train on data in the FDDB dataset. In the unrestricted mode you can train on any data you like so long as it doesn't include images from FDDB. I ran the 10-fold cross-validation version of the FDDB challenge. This means I trained 10 CNN face detectors, each on 9 folds and tested on the held out 10th. I did not perform any hyper parameter tuning. Then I ran the results through the FDDB evaluation software and got this plot:
The X axis is the number of false alarms produced over the entire 2845 image dataset. The Y axis is recall, i.e. the fraction of faces found by the detector. The green curve is the new dlib detector, which in this mode only gets about 4600 faces to train on. The red curve is the old Viola Jones detector which is still popular (although it shouldn't be, obviously). Most interestingly, the blue curve is a state-of-the-art result from the paper Face Detection with the Faster R-CNN, published only 4 months ago. In that paper, they train their detector on the very large WIDER dataset, which consists of 159,424 faces, and arguably get worse results on FDDB than the dlib detector trained on only 4600 faces.
As another test, I created the dog hipsterizer, which I made a post about a few days ago. The hipsterizer used the exact same code and parameter settings to train a dog head detector. The only difference was the training data consisted in 9240 dog heads instead of human faces. That produced the very high quality models used in the hipsterizer. So now we can automatically create fantastic images such as this one :)
![]() |
Barkhaus dogs looking fancy |
As one last test of the new CNN MMOD tool I made a dataset of 6975 faces. This dataset is a collection of face images selected from many publicly available datasets (excluding the FDDB dataset). In particular, there are images from ImageNet, AFLW, Pascal VOC, the VGG dataset, WIDER, and face scrub. Unlike FDDB, this new dataset contains faces in a wide range of poses rather than consisting of mostly front facing shots. To give you an idea of what it looks like, here are all the faces in the dataset tightly cropped and tiled into one big image:
Using the new dlib tooling I trained a CNN on this dataset using the same exact code and parameter settings as used by the dog hipsterizer and previous FDDB experiment. If you want to run that CNN on your own images you can use this example program. I tested this CNN on FDDB's unrestricted protocol and found that it has a recall of 0.879134, which is quite good. However, it produced 90 false alarms. Which sounds bad, until you look at them and find that it's finding labeling errors in FDDB. The following image shows all the "false alarms" it outputs on FDDB. All but one of them are actually faces.
Finally, to give you a more visceral idea of the difference in capability between the new CNN detector and the old HOG detector, here are a few images where I ran dlib's default HOG face detector (which is actually 5 HOG models) and the new CNN face detector. The red boxes are CNN detections and blue boxes are from the older HOG detector. While the HOG detector does an excellent job on easy faces looking at the camera, you can see that the CNN is way better at handling not just the easy cases but all faces in general. And yes, I ran the HOG detector on all the images, it's just that it fails to find any faces in some of them.
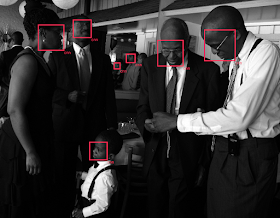
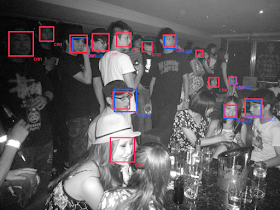
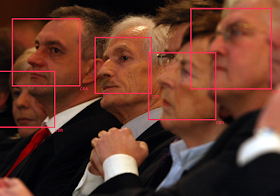
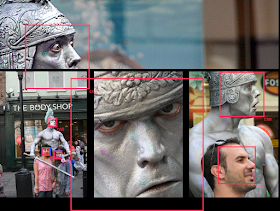
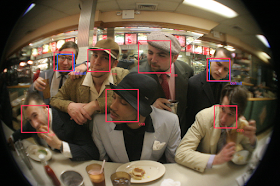